Comparison of State-of-the-Art Dynamic Machine Learning Methods for MPC of Coal-Fired Utility Generator Performance
Published: January 21, 2021
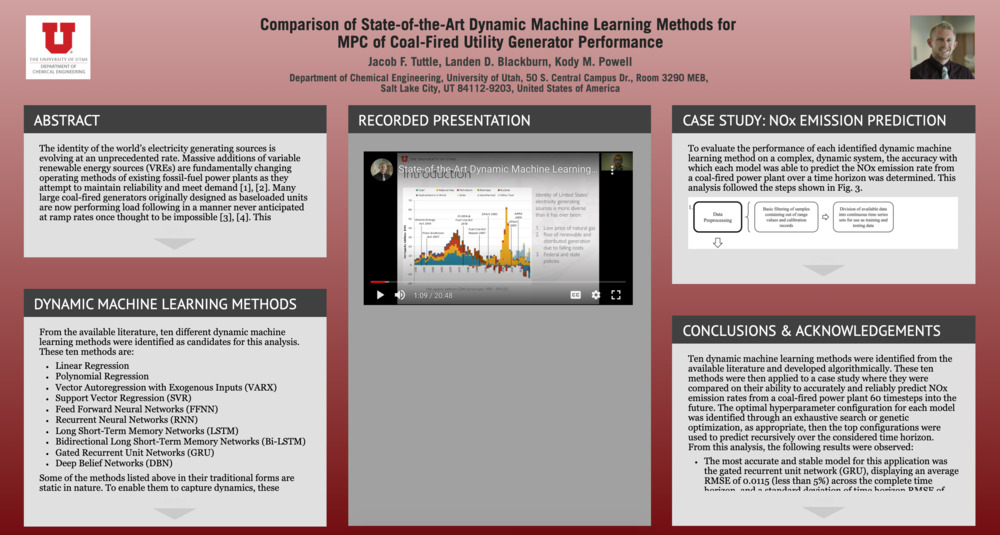
The identity of the world’s electricity generating sources is evolving at a rapid rate. Significant additions of variable renewable energy sources (VREs) are fundamentally changing operating methods of existing fossil-fuel power plants.
Real-time artificial intelligence optimization systems have been shown to consistently reduce emission rates of fossil-fuel power plants. In this presentation, Comparison of State-of-the-Art Dynamic Machine Learning Methods for MPC of Coal-Fired Utility Generator Performance, ten different dynamic machine learning methods are analyzed and applied to a case study to determine the optimal model for enhanced coal-fired utility generator performance. Each method was compared on their ability to accurately and reliably predict NOx emission rates from a coal-fired power plant 60 timesteps into the future.
The most accurate and stable model for this application was the gated recurrent unit network (GRU), displaying an average RMSE of less than 5% across the complete time horizon, and a standard deviation of time horizon RMSE of less than 1%.
See how the other models stacked up and watch a full presentation on how accurate each method was at predicting the NOx emission rate.
While operational support costs of using predictive machine learning models can be costly, the Griffin AI toolkit can be paired with the Power Plant Initiative, a maintenance program that reduces labor and maintenance costs. For a limited time save 80% on the Power Plant Initiative program with only a one year commitment or replace your existing system at a discounted installation rate. Try the Griffin AI Toolkit today or contact us to take advantage of the cost savings.